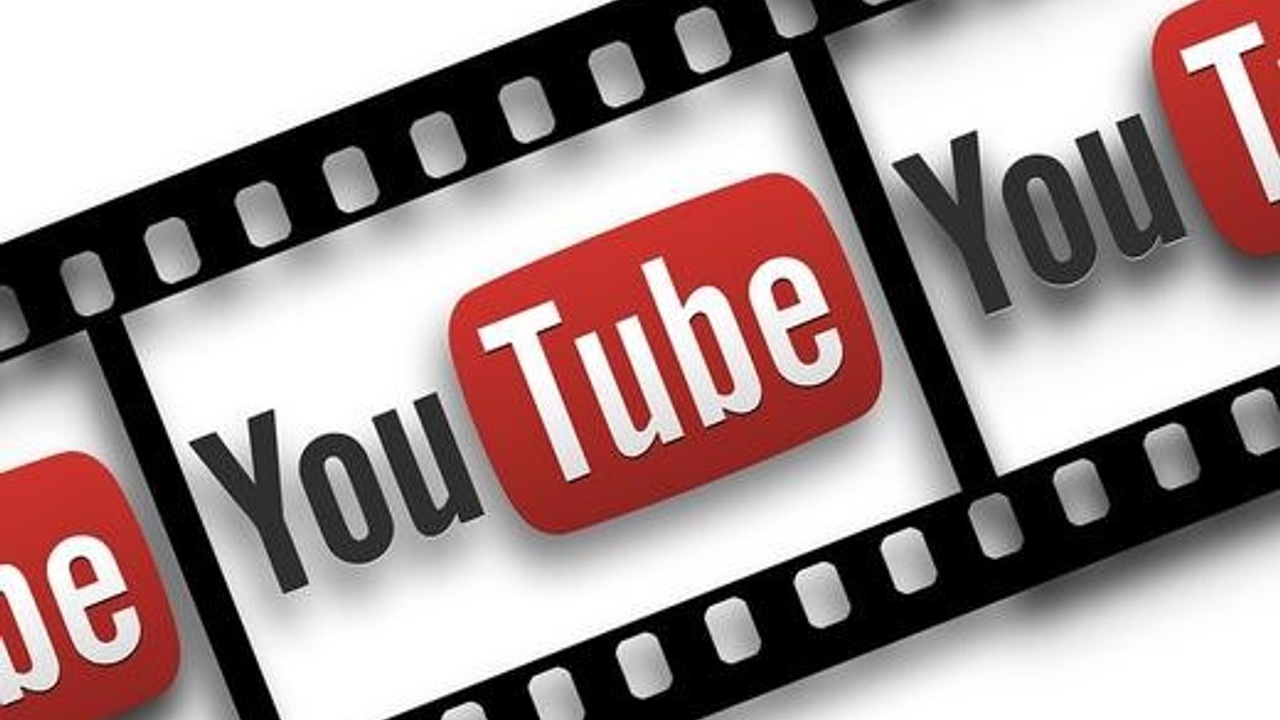
YouTube is a powerhouse of content, SEO, and marketing. However, with a parent company like Google, it is hard for the company to be anything less than an SEO prodigy. In fact, seventy percent of views on YouTube come from the recommendations, initiated by artificial intelligence.
The YouTube Video Recommendation System, primarily authored by five Google employees, explains the SEO algorithm behind this AI recommendation structure. These tips are a product of this paper, to help influencers approach their own YouTube video SEO correctly.
The Importance of Metadata
The AI system that YouTube uses to recognize appropriate recommendations are fueled by metadata. Similarities in metadata, throughout the platform, help tell the platform which videos belong in which categories. The system has reached a point where it is able to intelligently decipher metadata and captions for videos. Yet, that is not introducing any different information into the equation. There needs to be a human touch for metadata to be truly helpful. Keep a keen eye on these seven areas of a video post where metadata is crucial:
- Title: The title is amply important to the whole of the video. The title is the hook that draws people in. Therefore, the keywords that are used in the video should reflect this title. Yet, it should also be interesting. Even to an untrained eye, a keyword-stuffed title looks desperate, regardless of the video’s value.
- Description: The description of the video is an expansion of the title. This piece of content should be 250 words long and use as many keywords as possible. (Although, remember to also make it appealing.) Variations of keywords are okay and can be used as a secondary failsafe. Yet, using the actual keywords in the description are metadata gold. Remember, the more metadata the influencer gives the system to work with, the more accurate the results will be.
- Tags: Tags are a thing of the past for true content creation and search engine placement. Yet, tags are still vital to YouTube metadata. Therefore, tagging the keywords and variations of those keywords are essential. Plus, make sure to tag any other YouTubers that are mentioned in the video.
- Playlists: Playlists are important for a YouTube channel because it is a hyperextension of related content, within the influencer’s channel. This helps the metadata and the AI recommendation system find relatable information. Playlists can help keep visitors engaged in the influencer’s content which will also help boost video recommendations.
- Thumbnail: The thumbnail for the video is also important because it gets people interested in the video, much like the title. Likely, the thumbnail is the first or second impression a potential viewer is going to have of the video. Therefore, it plays a large role in garnering video views.
- Closed Captions: Providing a full transcript within the metadata of the video will help with accuracy. It will also help boost recommendations. The YouTube system can transcribe most videos, it is better to have the transcription included for accuracy.
- File Name: The file name is not the most important aspect of metadata. The file name has become less important over time. Yet, having keywords in the file name will only help the resources YouTube gathers from the video.
Utilizing Video Data
Video data is rising in importance. As technology advances and AI programs can pick more information out of the videos themselves, video data is vital. That is why it is important to speak the keywords throughout the video. That way, the AI transcriber can easily confirm the correct captions.
Additionally, Google has developed Cloud Video Intelligence API which is ‘learning’ to recognize images, as well as tags and texts. This technology is still in its infancy but is growing within the industry daily. The result of this API has already proven unprecedented in the advancement of garnering important information from raw video data.
Therefore, including images and videos within projects, which reference keywords will help this API learn. This will also help the recommendation score as the technology becomes more advanced.
Understanding User Data
User data is exactly what it sounds like; it is how users react to the content that is posted on an influencer’s channel. That is what the breakdowns in viewer times portrays on the YouTube Creator Studio, among other aspects of interaction. While influencers cannot exactly control this portion of the SEO for their channel, understanding it will give them insight.
The YouTube Recommendation paper explains user data as it relates to two different categories:
Explicit: This includes the action taken on the part of the users. This includes the number of likes a video receives and the number of subscriptions and influencer has to their channels.
Implicit: This category is mainly dealing with watch time. If there is no other action taken on the video, that user data is considered implicit. The paper notes that this category does not help to decipher whether the user enjoyed the video. The investment of time is not as heavily regarded as explicit actions.
That is why it is more important as an influencer, to encourage viewers to engage in explicit interactions. This is the reason why YouTubers ask their viewers outright to like and subscribe. However, implicit data does contribute to overall SEO. Therefore, it is still important to create videos which will garner quantifiable implicit user data.
All audience retention is important. As a channel grows, influencers should weed through their collection of videos and remove those that have poor audience retention. However, before removing videos, influencers should try to figure out why those videos did poorly.
The Power of Co-Visitation
Co-Visitation is the fundamental building block of YouTube’s recommendation machine. This is how viewers are introduced to other influencers, via similar videos. Watching one influencer’s playlist is nice. However, if someone enjoys videos of bird watching, it stands to reason they will enjoy many different bird watching enthusiasts. Therefore, the algorithm adapts to include co-visitation videos, from different influencers, with similar content.
However, the AI system also takes this a step further. Similar videos are not always so linear. Instead of simply having bird-watching videos, there might be other influencers who enjoy nature cued into this list. That is because the videos that are linked as similar are defined as videos that the user is more likely to watch after seeing the initial video. That does not always mean that the content is identical.
Co-visitation is devised by deciding how many times the videos were watched within a certain period. If the same videos were watched within a twenty-four-hour period, for example, they can be labeled co-visitation video candidates.
However, the popularity of the video and the influencer is also considered. The relatedness score is decided, ultimately by factoring co-visitation, watch time, and video metadata.
This means that influencers not only need to get many people to watch videos, they also need people to watch other related videos within a certain timeframe. Here are the best ways to accomplish this feat:
- Creating response videos in a timely fashion
- Publishing videos on platforms that is known for sending traffic to other viral videos
- Targeting keywords acutely and even pinpointing another video in the keywords
- Targeting a specific YouTuber in created videos
- Promoting viewers to watch more of the videos on the channel
User Personalization Recommendations
While the basics of co-visitation is the foundation of the YouTube recommendation system, there are also personalization factors accounted for. This is what gives each user a unique YouTube recommendation experience.
This personalization is conceived by weighing videos watched by the amount of time the video was watched and the reaction. That is why it is important to get people to like the videos, in addition to watching them. This input helps the AI decipher the user’s type, style, and interest level of the videos they watch.
The result of this personalization is like an inverted triangle. The first set of videos with a high-relatedness score would should up as initial recommendations. After that, as the personalization system works, small differences in videos will be noted, making the recommendations more finite. This does not mean the number of video selections will be limited, though. Instead, the system will determine the type of videos the user wants far more accurately.
For influencers, this means that the video does not always need to have an ultra-high co-visitation count with the video in question. Instead, the video could have a high co-visitation count with a video that has a has a high co-visitation count with the video in question. It is akin to being a friend of a friend at a party. That friend will introduce you, which will result in you being seen with a high-profile individual.
Factors of Ranking High on YouTube
YouTube’s recommendation engine is an advanced system which was created to keep improving itself. Therefore, there is no finite recipe for success. Instead, influencers must continue to focus on the different factors that the YouTube paper identifies as important. These essential ranking entities are video quality, user specificity, and diversification.
- Video Quality: The quality of a video is always important, as it should be. It makes sense that a low-quality video would not do as well as a high-quality video. Yet, there are certain signals which give YouTube AI more information about the video quality. These signals include:
- User Ratings
- Comments
- Favoriting
- Shares
- Upload time
- View Count
- User Specificity: The specification of the videos is decided based on the individual user’s history. Therefore, there is a certain level of personalized building at play, which awards a relatedness score based on the specific user’s watch history. This makes the recommendation and quality results far more finite than simply basing the relation off the seed video.
- Diversity: Users likely do not want to watch what is essentially the same video, simply done by a different influencer. Therefore, videos that are determined to be too similar in nature are removed from the rankings. This makes room for a more meaningful, personalized selection.
In summation, the YouTube Recommendation Engine is the Rosetta Stone of connecting with users on the platform. Deciphering this system will drastically progress an influencer’s potential to be successful on the video site. After all, the system is all laid out. It takes a little bit of work and has a bit of a learning curve. Yet, it is undoubtedly worth the effort, resulting in stepping up your YouTube SEO marketing strategy.